Accounting
Why and How: Machine Learning
Machine Learning is still in its infancy and is making progress rapidly. Almost every provider to the profession is coding algorithms to learn about transactions. Every innovative company has a Machine Learning project to help learn and make very ...
Apr. 16, 2018
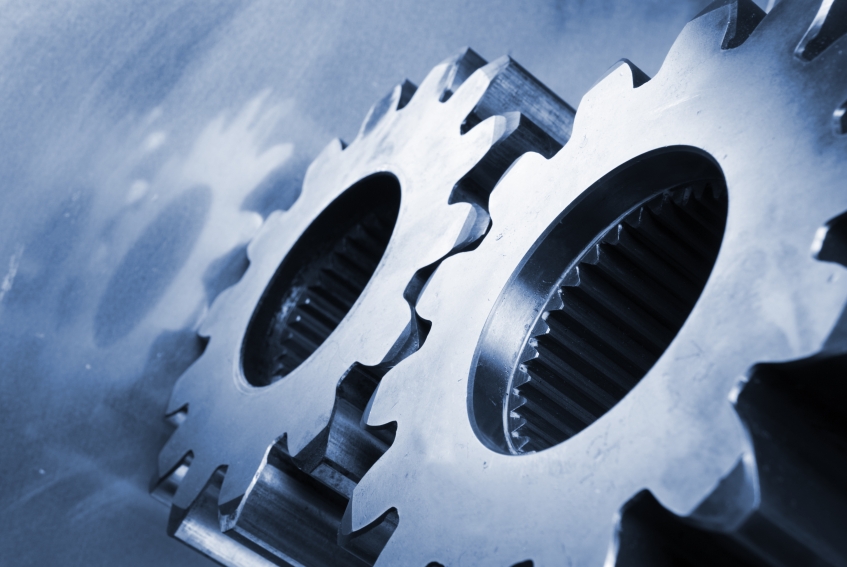
A Top Technology Initiative Article. From the April 2018 Issue.
Many us like to think that we are consistent, but not predictably boring. One thing I enjoy about technology is that it never stays the same. I’m sure you feel the same way about practicing accounting: things are never the same. While the practice of accounting is often driven by regulations and laws, technology is obsessively driven by change. As stated in prior columns, technology can be used for good or bad, but the developers of the technology are typically building their products because they are trying to do what no one else has done with technology while solving a problem that they have creatively seen.
I have a fairly complete road map of many topics to discuss with you and I’m sure we’ll take a few detours along the way based on new technology announcements. I’ve not seen this road map developed by anyone else, and I’m still marking out where there are luxury spas to enjoy and dens of thieves to avoid. When the map is complete and accurate enough, I’ll share it in a future column. But I have a vision of where we are going, and why, and these emerging technologies are driving a fourth industrial revolution. The changes over the next five to ten years will have a much longer-term impact that the development of the personal computer, smartphone or SaaS applications. That may not seem possible, but farther along, you’ll understand why. This third column in the series on emerging technology will discuss Machine Learning, one of the most interesting and useful emerging technologies. Wikipedia defines Machine Learning in an interesting, fact-filled way:
“Machine learning is a field of computer science that gives computer systems the ability to “learn” (i.e. progressively improve performance on a specific task) with data, without being explicitly programmed.[1] The name Machine learning was coined in 1959 by Arthur Samuel.[2] Evolved from the study of pattern recognition and computational learning theory in artificial intelligence,[3] Machine Learning explores the study and construction of algorithms that can learn from and make predictions on data[4] – such algorithms overcome following strictly static program instructions by making data-driven predictions or decisions,[5]:2 through building a model from sample inputs. Machine learning is employed in a range of computing tasks where designing and programming explicit algorithms with good performance is difficult or infeasible; example applications include email filtering, detection of network intruders or malicious insiders working towards a data breach,[6] optical character recognition (OCR),[7] learning to rank, and computer vision. Machine learning is closely related to (and often overlaps with) computational statistics, which also focuses on prediction-making through the use of computers.”
Some of the terms like algorithms, computational learning theory and computational statistics may be as foreign to you as some accounting terms were to you when you were just beginning school, but the key principle is that developers and computer scientists are trying to teach computers to learn on their own. Consider for a moment the capabilities of computers or other technologies like self-driving cars if they could learn tasks on their own. This may sound like a daydream, but real work is being done and real progress on solving real world problems is being made. (Really!?!) A few vendors to the profession truly have Machine Learning working and others are throwing the term around for sales purposes just like they have with cloud computing, artificial intelligence or “ease of use” before that.
Why?
As humans, we learn every day. Scientists tell us, as well as through our own observations, that we learn the most rapidly when we are younger. We know less, everything is new, and we have the unending question of why? As we age, some professionals slow down the amount that they learn. Others enjoy continuous learning, picking up and applying new information while expanding on the context of what they already know. An old saying reflects on how some people have 20 years of experience, and others have 1 year of experience repeated 20 times.
Machines can be programmed to have the curiosity of a four-year-old perpetually asking why, and then learning from the answer developing 20 years of experience if a few minutes or hours based on the rate they can learn. Machines will make some dumb mistakes because they don’t have our human logic, but this attribute of “judgment” is getting better, too. The two major tricks to get Machine Learning right is to 1) have the right sample data, and 2) have the right algorithm. Machine learning can be accelerated when it is run on special hardware. Although I’m looking for facts to support these observations, reports are that it takes from 10,000 to 1,000,000 records to for Machine Learning to work properly. While I’m sure there are many more, I reviewed 15 different algorithms and the approaches being used to develop Machine Learning algorithms. The most interesting to me was the neural network, Bayesian and clustering approaches. Digging into these algorithms reminded me of why Dr. Bob Spencer and I had computer science degrees and enjoyed our geek speak and analysis of the technologies.
Article continues online at: www.CPAPracticeAdvisor.com/12403864